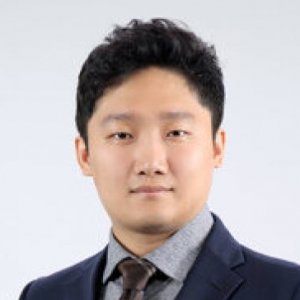
Martin Kang, Ph.D.
Assistant Professor of Information Systems and Business Analytics, College of Business Administration
- Los Angeles CA UNITED STATES
Biography
Education
Korea University Business School
Ph.D.
Business Analytics and IS
2016
Milwaukee School of Engineering
B.S.
Information Systems and Industrial Engineering
2010
Social
Areas of Expertise
Articles
The effects of government labour intervention on firm performance and innovation (Forthcoming)
Applied EconomicsThe government sets legal limits on the number of working hours to minimize the negative effects of long working hours. Reducing legal working hours increases employee life satisfaction by decreasing mental and physical health problems. However, reducing legal working hours may decrease the firm’s future investment opportunities, which reduces the firm’s competitiveness. This study uses a quasi-experimental design to explore the negative effects of reducing legal working hours on firms. Specifically, we build a difference-in-differences (DID) model to explore the negative effects of reducing legal working hours on a firm’s ROI and innovation. We find reducing legal working hours decreases a firm’s ROI and innovation.
A Method Framework for Identifying Digital Resource Clusters in Software Ecosystems (Forthcoming)
Decision Support SystemsMartin Kang, Gary Templeton, Ted Lee, and Sungyong Um
A popular form of modern software development involves co-creation and sharing of digital resources (e.g., API and SDK) by third-party developers in software ecosystems. In doing so, digital resources, software development projects, and developer organizations are networked together and form digital resource clusters (DRCs), which can be closely or loosely related. Management science and innovation research has focused on how organizations use DRCs to innovate their software products and services. However, the network structures of software ecosystems are spatiotemporally complex since digital resources are transitively, heterogeneously, and temporally related. In existing literature, the extent of spatiotemporal complexity has impeded the empirical identification of DRCs. Our research devises a method framework consisting of two steps toward identifying DRCs using machine learning, which we found to be well suited to representing the spatiotemporal characteristics of networked digital resources. First, we devise a spatiotemporal network embedding method that learns and represents temporal, transitive, and heterogeneous networks of software ecosystems. Second, we devise a clustering method that identifies DRCs using the output of our embedding method as input. The performance test experiment results show that our devised method framework is superior to existing conventional methods at identifying DRCs.
Development of a Method Framework to Predict Network Structure Dynamics in Digital Platform: Empirical Experiment based on API Network (Forthcoming)
Knowledge Based SystemsMartin Kang, Ted Lee, Austin Kwak, Sungyong Um
Digital ecosystems reinforce the commercial achievements of digital innovations, providing organizations with platforms to implement digital products by sharing, co-developing, and using application programming interfaces (APIs) as digital resources. The use of APIs in digital ecosystems formulates dynamic API networks that evolve with the emergence of APIs and their updates. API network dynamics are associated with disruptive technology, heterogeneous networks, product and service innovation, and entrepreneurial success. However, methods for measuring and predicting API network dynamics have not been developed. We developed a framework for measuring and predicting the API network dynamics generated by APIs. To develop the abovementioned framework, we invented three network embeddings that could represent and measure API network dynamics and a prediction model based on a deep learning approach that could forecast API network dynamics. We conducted multiple experiments to assess the performance and usability of our method framework, and the results consistently demonstrate that our developed approach surpasses existing methods.
Vulnerability diffusions in software product networks
Journal of Operations ManagementMartin Kang, Gary Templeton, and Sungyong Um
2023-07-16
During software product development, the combination of digital resources (such as application programming interfaces and software development kits) establishes loose and tight edges between nodes, which form a software product network (SPN). These edges serve as observable conduits that may help practitioners and researchers better understand how vulnerabilities diffuse through SPNs. We apply network theory to analyze data from over 12 years of records extracted from the National Vulnerability Database. We contribute novel measures established using machine learning to gauge the properties influencing vulnerability diffusion within an SPN. We observed an SPN having a discernable shape that changed over time via network updates. We propose hypotheses and find empirical evidence that vulnerability diffusion is influenced by edge dynamics, developer responses, and their interaction. Implications for practice are that increased developer responses reduce software vulnerability diffusion attributed to edge dynamics.
Firm performance and information security technology intellectual property
Technological Forecasting and Social Change 181Martin Kang, Andrew Miller, James Jang, and Horim Kim
2022-08-01
The appropriate valuation of information security (InfoSec) intellectual property (IP) is of interest to both business scholars and investors. This topic is important because InfoSec IP is a complementary knowledge resource that can complement a firm's existing and new products, services, business models, and operations. This paper attempts to understand the marginal effects of having additional InfoSec IP on a firm's performance in terms of return on investment and market capitalization.
Development of methods for identifying an appropriate benchmarking peer to establish information security policy
Expert Systems with Applications 201Martin Kang, Ted Lee, Anat Hovav, Sungyong Um, and Horim Kim
2022-04-11
Benchmarking methodology provides organizations with appropriate information security policy. However, selecting an appropriate organization as a benchmarking peer can be a challenge due to firms’ heterogeneous implementation and usage of information systems. Our goal is to develop and propose methods to appropriately identify a benchmarking peer organization by incorporating machine learning methods and mathematics set theory. We incorporate vague soft set, entropy, dynamic time warping, and Gaussian process. We use log data from information security management systems in multiple companies to validate our methods. Our experimental results indicate that the combined use of Gaussian process, vague soft set, and dynamic time warping can be more effective in identifying an appropriate benchmarking peer than conventional machine learning methods.
Regression imputation optimizing sample size and emulation: Demonstrations and comparisons to prominent methods
Decision Support SystemsTempleton, Gary F., Martin Kang, and Nargess Tahmasbi
2021-12-15
Missing input values weaken the ability of information systems (IS) researchers to make calculations, thereby reducing effective sample sizes and statistical power. Such technical problems with data cascade into scientific limitations resulting in the neglect of social and economic issues. Therefore, extensive missing values in data forces researchers to make crucial decisions, such as whether to impute and if so, what strategy to use. This study presents a single imputation approach that integrates and extends best practices for mitigating the effects of missing values. Using an array of missing value situations, we illustrate the Regression Imputation Optimizing Sample Size and Emulation (RIOSSE) method.
Benchmarking Methodology for Information Security Policy (BMISP): Artifact Development and Evaluation
Information Systems FrontiersMartin Kang and Anat Hovav
2018-05-07
The benchmarking of information security policies has two challenges. Organizations are reluctant to share data regarding information security and no two organizations are identical. In this paper, we attempt to propose an artifact for a benchmarking method of information security policy, which can resolve the above challenges. We employ design science methodology, activity theory and international standards to design the artifact as a proof of concept. The artifact facilitates the implementation of efficient information security policies. Organizations can utilize the artifact to analyze and benchmark information security policies. We illustrate the completeness and reliability of the artifact through a case study using information security policies from six companies.
Business model innovation through value delivery differentiation: Multiple case studies
Journal of Science and TechnologyMartin Kang and Jaeyoung Kim
2015-09-28
There are many highlights on value creation and value capture in the field of business model research since these are traditionally considered as core components of a business model. However, the concept of value delivery is open to question what does value delivery play a role in the concept of business model. In our research, we examined the roles of value delivery in a business model. Using multiple case studies of firms and its business model, we found evidence that differentiation of value delivery would impact business model innovation. This research proposes a theoretical framework for organizational capabilities to achieve successful value delivery differentiation. The results have implications for business model establishment practices.